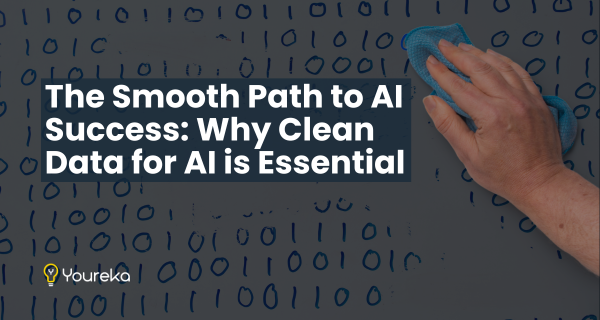
The promise of AI is at your fingertips—greater efficiency, sharper insights, and the ability to make data-driven decisions on the spot. But there’s a catch: AI only works as well as the data you feed it. For many operations, collecting useful and accurate data is harder than it sounds.
Bad data costs the U.S. economy over $3 trillion annually. For individual companies, the impact is significant—both in missed opportunities and inefficiencies. Gartner estimates that poor data quality costs the average U.S. company approximately $15 million each year. With the availability of AI, the stakes to have clean data have never been higher.
From energy companies trying to track equipment in the field to manufacturers seeking real-time metrics on production lines, the need for clean, consistent data is critical. Yet, for most organizations, the path to harnessing AI’s potential often gets bogged down by messy, inconsistent data that just doesn’t work.
Luckily, there are tools available to make data collection smoother and more reliable.
Why Data Collection Feels So Hard
With every new customer interaction, field inspection, or compliance report, companies generate a mountain of data. But more data doesn’t mean better data. Data collection challenges usually boil down to a few key issues:
1. Inconsistent Formats: When field reports, inspection logs, and customer data come in different formats, AI tools can’t effectively process the information.
2. Data Gaps: Missing data creates “holes” that make AI predictions unreliable. These gaps often stem from field data collection challenges, especially in offline environments where connectivity issues disrupt workflows.
3. Siloed Systems: Often, data exists in separate systems that don’t communicate, leading to fragmented or duplicated information.
These challenges don’t just slow down AI implementation; they also prevent AI from delivering the real-time insights it’s capable of.
Why Clean Data is Essential for AI Success
The quality of your data directly impacts the accuracy and reliability of your AI models. Here’s why clean data is critical:
– Performance and Accuracy: Clean data allows AI to generate precise, actionable insights. Conversely, poor data quality is a common reason AI projects fail. For example, Shell relied on Youreka’s tools to ensure consistent, accurate data collection in some of the most demanding environments, enabling faster decision-making and reducing operational inefficiencies. When data quality is prioritized, companies can unlock the full potential of their AI initiatives, driving smarter, more reliable outcomes.
– Enhanced Decision-Making: Clean, accurate data helps AI recognize genuine patterns, which empowers your organization to make informed decisions quickly. Without clean data, AI models may present skewed insights that lead to misguided strategies and operational inefficiencies.
Practical Steps for Improving Data Collection
Improving data quality may sound daunting, but you can achieve substantial gains by taking a few actionable steps. Here’s how:
1. Standardize Data Collection Processes
- Dynamic forms like Youreka’s ensure consistent, efficient data capture, even offline. This eliminates gaps and enables seamless operations in remote areas, delivering faster, more reliable AI insights.
2. Automate Data Collection Setup
- Use Youreka’s Template Copilot to quickly create custom form templates from simple prompts or PDFs, ready for deployment across mobile devices. This AI-powered tool streamlines form template creation, allowing admins to generate, refine, and publish forms in minutes. By making data collection workflows faster to set up and easier to scale, Template Copilot helps teams capture consistent data while reducing setup time and onboarding costs.
3. Ensure Near Real-Time Synchronization Across Systems
- Use solutions with offline capabilities so that even field teams in remote areas can collect accurate data. Youreka, for instance, enables data capture that stays reliable even when teams are offline. Once reconnected, data syncs seamlessly, ensuring no information is lost.
4. Remove Duplicates and Inconsistencies
- Establish a regular cadence for data cleansing. Automated deduplication tools can identify and remove duplicate entries across systems, helping prevent data clutter that could undermine AI models.
AI Use Cases: Clean Data Drives AI Results
Companies that prioritize clean data see far better AI outcomes. Here are some real-world examples:
– Energy Sector: Energy & Utilities operations use AI-powered tools to monitor field assets for predictive maintenance. Accurate, clean data captured through platforms like Youreka allows for real-time equipment assessments, helping companies reduce downtime and improve compliance records.
– Construction Industry: In construction, clean data enables real-time project tracking, safety compliance, and resource management. Using Youreka’s offline capabilities, teams capture accurate on-site data (photos, QR codes, safety checks) that syncs to Salesforce, allowing AI to optimize schedules and identify potential delays. This reduces risks, prevents errors, and keeps projects on track.
– Field Service Operations: In field service, AI tools rely on precise data to automate workflows and anticipate maintenance needs. Youreka’s smart forms guide technicians in collecting essential data, which AI then analyzes for predictive maintenance and service optimization. This reduces downtime, improves customer satisfaction, and streamlines equipment management.
Future Implications and Opportunities
By laying a solid foundation of clean data, organizations can take full advantage of AI’s scalability, accuracy, and predictive power. Clean data doesn’t just prepare your organization for the present; it also opens up future opportunities. As your data quality improves, AI models become increasingly adept at identifying trends and offering advanced insights, positioning your organization to stay agile and proactive in an evolving landscape.
Making Data Work for Your AI Goals
Building clean data practices is the first step toward successful AI implementation. Solutions like Youreka’s Template Copilot and Guided Procedures integrate seamlessly into existing workflows, ensuring your data is accurate, consistent, and AI-ready. Interested in learning more about data collection for AI?
See how Youreka works to help you achieve smooth, scalable AI operations.